Error-Free Underwriting Automation
UWDataHub Data Standardization and Request Filtering Tool
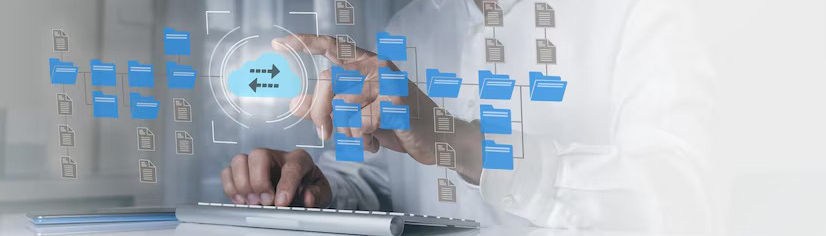
Welcome back to
“The Underwriter’s Guide to Efficiency: Everyday Wins with UWDataHub.”
In our first post, we explored how UWDataHub streamlines data collection through automation. Today, we’ll delve into how UWDataHub’s data validation tools ensure error-free underwriting, improving the accuracy of your risk assessments and pricing decisions.
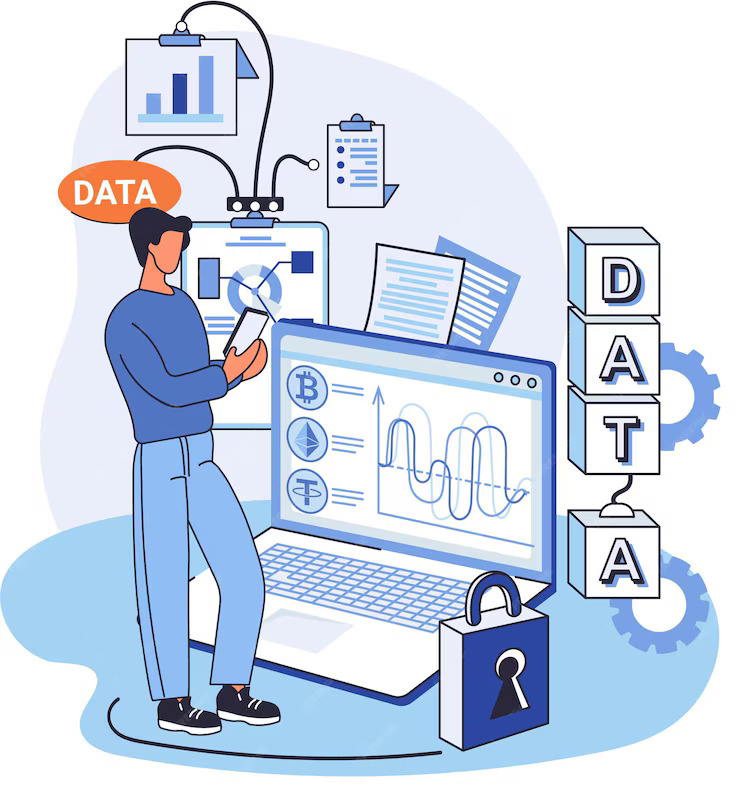
The Challenge
Impact of Data Errors on Risk Assessments and Pricing
As an underwriter, you rely heavily on data to make informed decisions. However, data errors can significantly impact your risk assessments and pricing, leading to inaccurate quotes, potential losses, and compromised client trust. Common data errors include incorrect client information, missing data fields, outdated records, and inconsistent data formats. These issues can result in:
Inaccurate Risk Assessments
Erroneous data can lead to misjudging the level of risk associated with a policy, potentially resulting in underpricing or overpricing.
Inefficiencies
Time spent identifying and correcting data errors delays the underwriting process, affecting productivity and turnaround times.
Increased Costs
Incorrect pricing can lead to financial losses, either through undercharging and reduced profitability or overcharging and losing clients.
The Solution
UWDataHub’s Data Standardization and Request Filtering Tool
UWDataHub Underwriting Workbench addresses these challenges with it’s robust Data Standardization and Request Filtering Tool, the AI-Based SmartExtractor™ and SmartRules™ Engine, ensuring that the data you work with is accurate, consistent, and reliable. Here’s how UWDataHub Underwriting Workbench can transform your underwriting process:
AI-Based SmartExtractor™ for Data Standardization
Standardized Data Formats: AI-Based SmartExtractor™ ensures that data from various sources is standardized to your format without requiring additional manual work, making all information compatible and easily analyzable. This consistency reduces errors and enhances data quality across the board. It also ensures that the data you work with is consistent, and reliable, promptly giving alerts if any discrepancies are found.
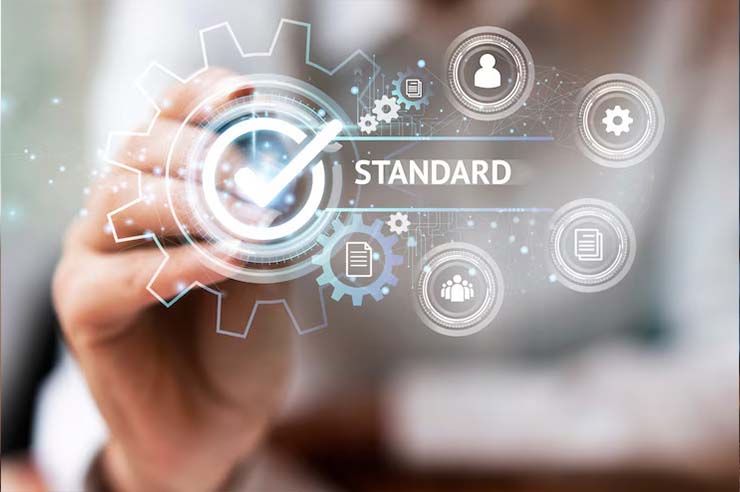
How It Works
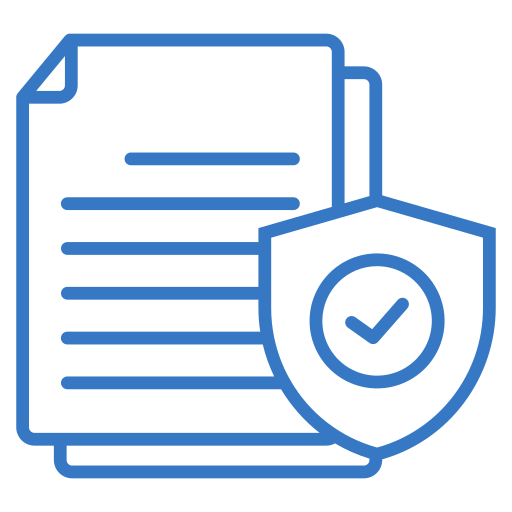
Step 1
Automated Data Validation
As data is collected, UWDataHub’s AI-Based SmartExtractor™ standardizes data formats, ensuring all information is compatible and easily analyzable.
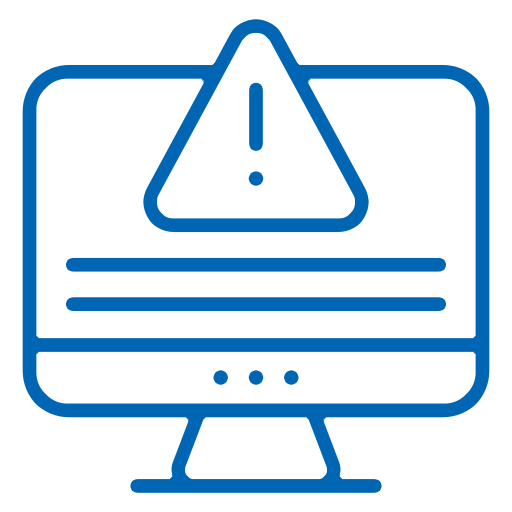
Step 2
Real-Time Error Alerts
When the system identifies a potential error, it generates a detailed alert, highlighting the specific issue that needs attention.
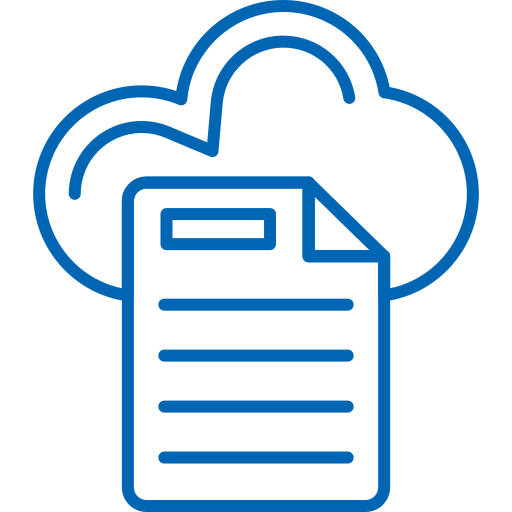
Step 3
Data Standardization
All data is formatted consistently, regardless of the source such as dates, gender, and zipcode, reducing the risk of format-related errors.
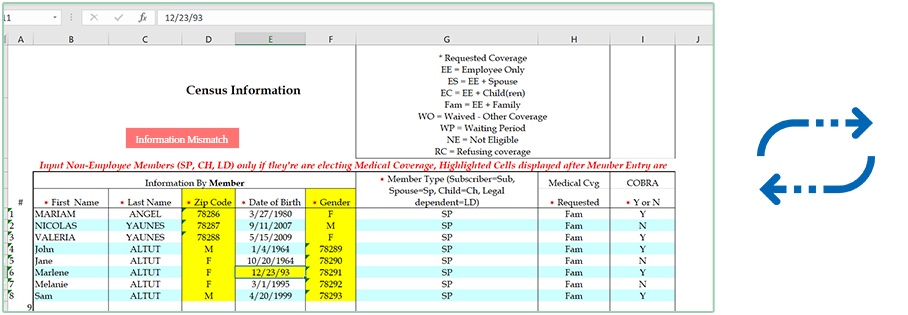
Examples of Specific Data Validation
To illustrate the effectiveness of UWDataHub’s data validation tools, consider the following examples:
Example 1:
Missing Data Fields
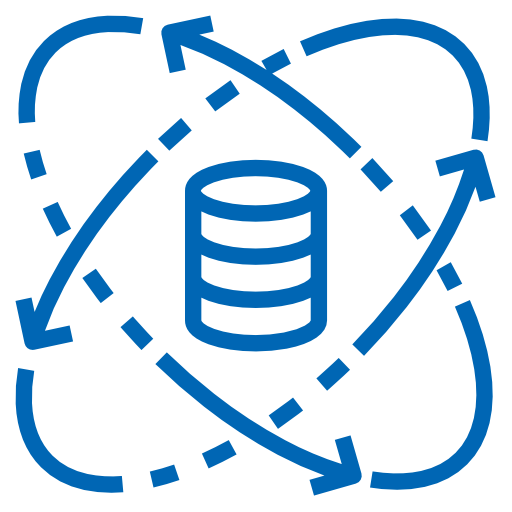
Problem: A new policy application is missing crucial zip code information, delaying the underwriting process.
Solution: UWDataHub checks for completeness, ensuring all required fields are filled out before the application proceeds to the next stage.
Example 2:
Inconsistent Data Formats
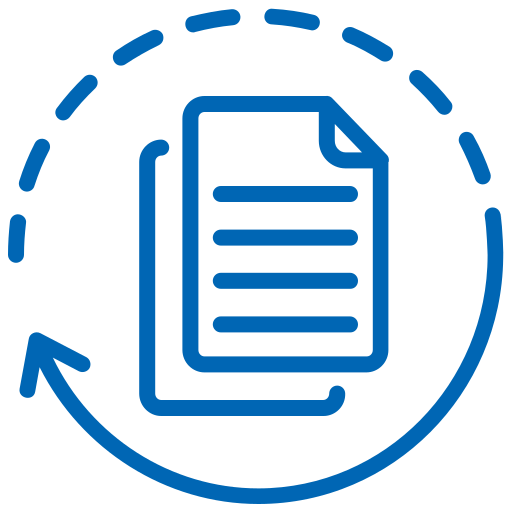
Problem: Figures are entered in different formats (e.g., MM/DD/YY vs. MM/DD/YYYY), causing confusion and errors in risk assessment.
Solution: UWDataHub standardizes the format of all data, ensuring consistency and clarity.
SmartRules™ Engine for Efficient Request Filtering
Efficient Filtering: By filtering incoming requests against predefined business rules and guidelines, the SmartRules™ Engine reduces the workload on underwriters, allowing them to focus on complex cases. SmartRules™ expands the opportunity for an underwriter to not only filter the risks but also highlights the essential parameters that influence a risk such as Industry, Area, Contribution strategy etc.
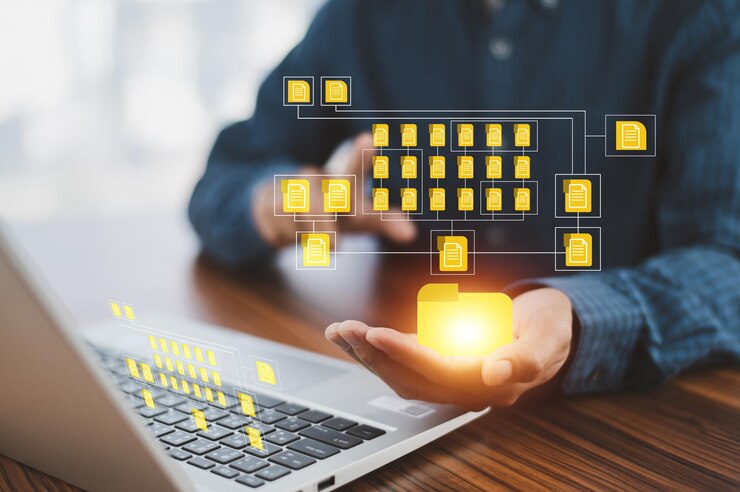
How It Works
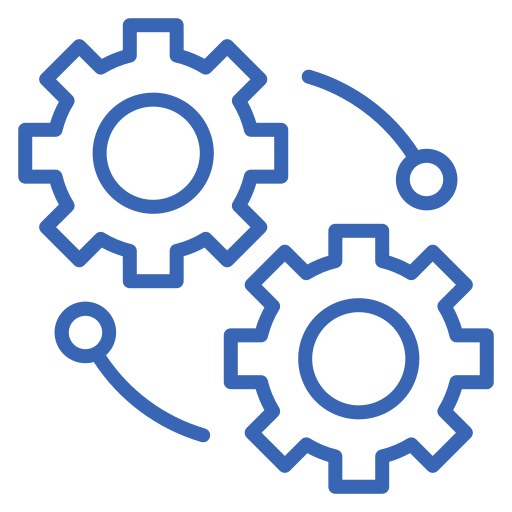
Step 1
Rule Settings
Access the rule settings to configure the filtering criteria.
-
- Path: SmartRules™ Engine
- Action: Create Underwriting rules for pre-approval popup.
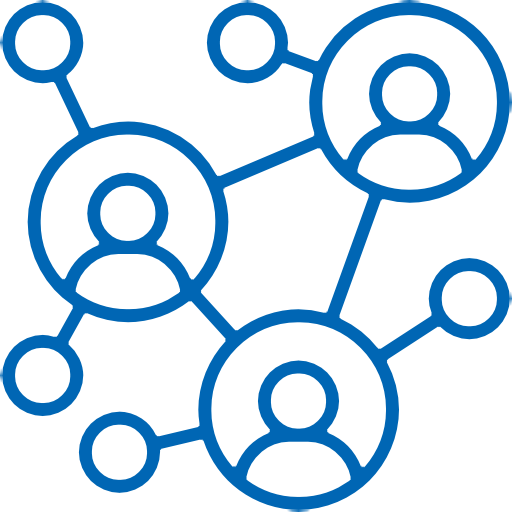
Step 2
Define Quote and Group Types
Select the appropriate quote and group types for the rules.
-
- Select Quote Type: Choose from the dropdown menu.
- Select Group Type: Choose the applicable group type.
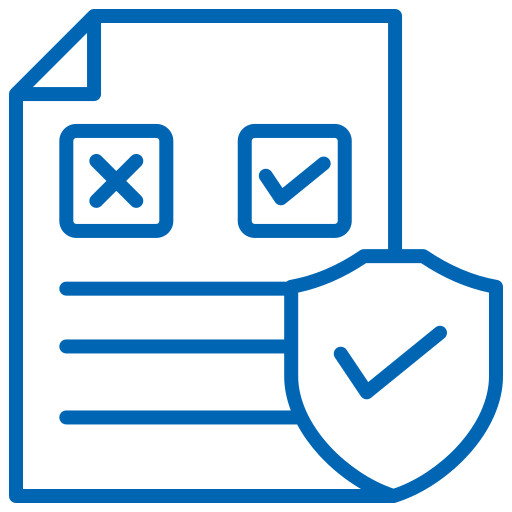
Step 3
Specific Rules (Customizable)
Toggle specific rules on/off to filter requests effectively. Example:
-
- Employee Location: Define acceptable percentage for employees outside certain states.
- SAIL Score: Set a maximum threshold for SAIL score.
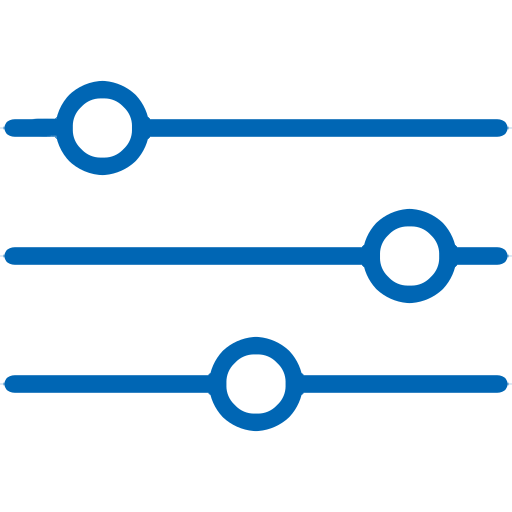
Step 4
Filtering Requests
Automatically filter incoming requests based on predefined rules.
-
- Process: Requests that meet all criteria are forwarded without the need for manual underwriting.
- Flagging: Requests failing any rule are flagged for manual underwriting or automatically rejected.
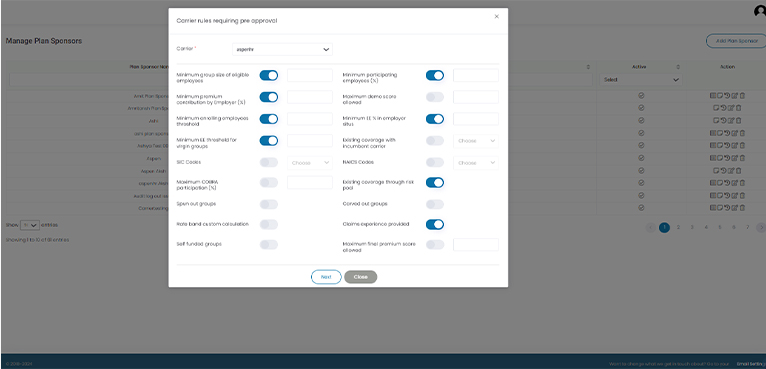
Examples of Specific Efficient Request Filtering
Example 1:
Employee Location-Based Filtering
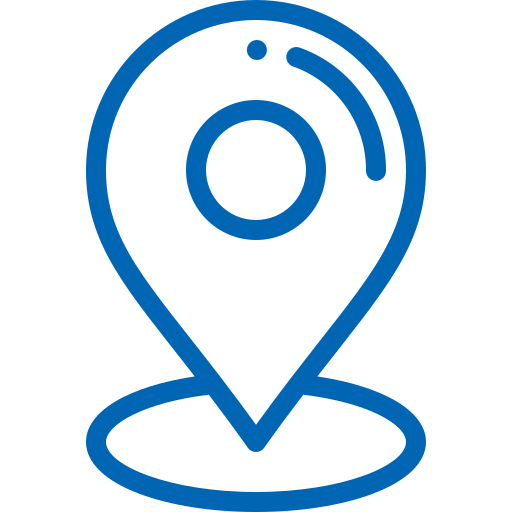
Rule: Enrolling employees outside Oregon must not exceed 50%.
Application: A quote request with 60% employees outside the specified state is flagged for review.
Outcome: Ensures compliance with location-based guidelines.
Example 2:
SAIL Score Threshold
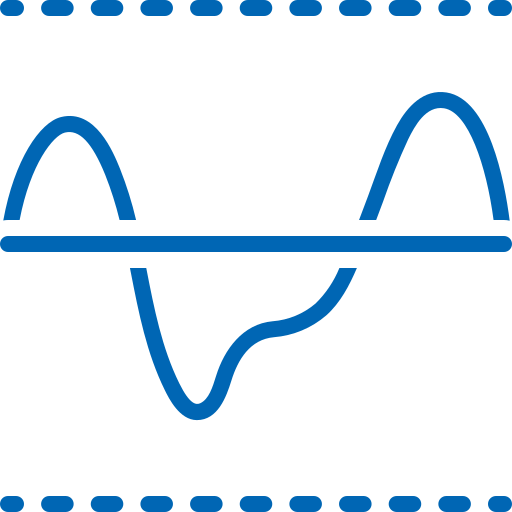
Rule: SAIL score must not exceed 70.
Application: A request with a SAIL score of 75 is automatically rejected.
Outcome: Filters out high-risk profiles, allowing underwriters to focus on manageable risks.
Example 3:
Minimum Group Size
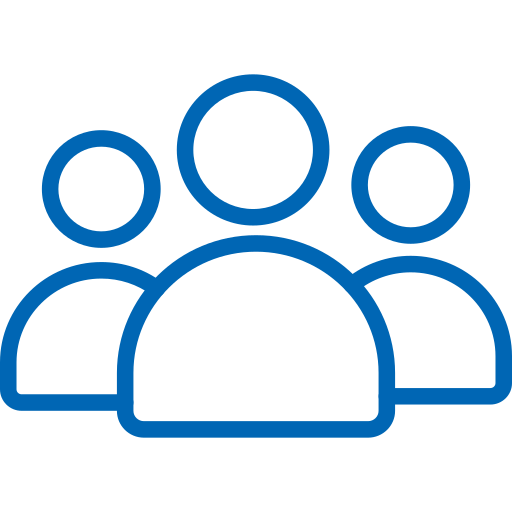
Rule: Group size must be at least 50 employees.
Application: A request with a group size of 30 employees is flagged.
Outcome: Ensures processing of requests with sufficient group size, enhancing efficiency.
Example 4:
Renewal Increase Cap
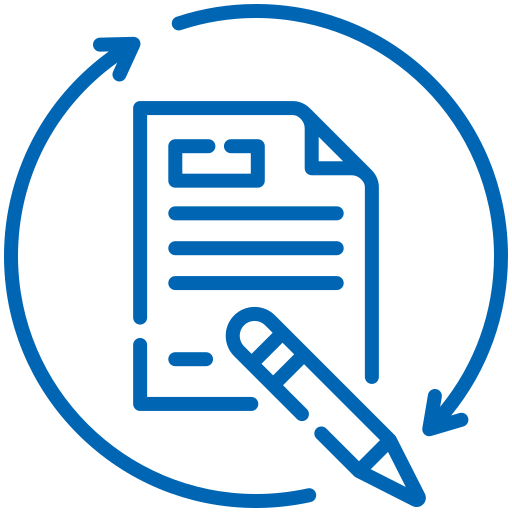
Rule: Renewal increase must not exceed 10%.
Application: A request proposing a 12% increase is rejected.
Outcome: Maintains compliance with business guidelines and avoids excessive renewal rate increases.
By following these structured steps and examples, the SmartRules™ Engine effectively streamlines the underwriting process, reduces manual workload, and enhances overall efficiency.
Conclusion
By utilizing UWDataHub’s data validation tools, including the SmartRules™ Engine, and standardizing data with SmartExtractor™, you can ensure that your underwriting data is reliable, and consistent. This not only improves the quality of your risk assessments and pricing decisions but also enhances overall efficiency and productivity. Stay tuned for our next blog post, where we’ll explore another feature of UWDataHub that simplifies your daily tasks and enhances your work experience.
Download The Guide
For a more comprehensive look at how UWDataHub Underwriting Workbench can transform your underwriting processes, download our free guide, “Digital-First Underwriting using UWDataHub Underwriting Workbench Underwriting Workbench.”
Stay tuned for the next post in our series, where we will dive deeper into how UWDataHub Underwriting Workbench helps underwriters streamline other key tasks and workflows.
Empowered Margins – Empowering Underwriting Excellence